An Alternative Application of Artificial Intelligence and Economics
The Digital Revolution, according to a quick Wikipedia search, is also considered to be the “Third Industrial Revolution”, implicitly referring to the changes brought about by the rapidly developing digital communication that marks the beginning of the so called “Information Age”.
As a Warwick Economics student of the early 2000’s, sometimes being referred to as a member of the “Millennials” generation, my fellow Students and I were no strangers to the sweeping changes that have come about from globally booming enterprises such as Amazon, Google, Blackberry and even Facebook. The opportunities that presented themselves appeared to be infinite, but where do Economists fit into the equation?
The necessity to make breakthroughs within AI is now more relevant than ever before, even for economics!
According to the career fairs at the time, the most typical positions offered to economics students and graduates were within the realms of research, academia, investment banking and business consulting. It appeared to me that economists were considered de-facto multi-talents, or so-called generalists, nevertheless with only a marginal likelihood of entering the technology sector. Fortunately (or unfortunately), the peak of the financial crisis of 2007 / 2008 tested this theory in such a way that banks and consultancies had to rationalize their hiring efforts, forcing graduates, like myself, to look beyond.
What technological aspect could an aspiring economist be more likely eager to explore? There are several, but my educated guess is that the most likely to change economic mechanics would be Artificial Intelligence. AI involves the development of computer systems that are able to perform tasks normally requiring human intelligence, such as i) perception, ii) recognition, iii) decision-making, and iv) translation, an aspect that was still considered only to be suitable for movies and video games in the 1990s and early 2000s. The necessity to make breakthroughs within AI is now more relevant than ever before, even for economics!
At this stage, many economics students would pinpoint to the obvious consideration of algorithmic trading. The primary ambition being to go beyond mechanical trading rules to also consider unusual parameters in order to exploit arbitrage opportunities by the means of mining through financial variables in the tiniest fraction of a second. Such techniques have continuously been enhanced to incorporate other inputs, which in effect incorporate volumes, market momentum and searches for cumulative indicators in i.e. Twitter to produce trading signals and act on them. Economics graduates would consider themselves lucky being able to incorporate their knowledge from Macroeconomics, Microeconomics and Econometrics to the designing of such multi-variable models that would produce statistically significant results. Recent evidence, as many of the graduates may have discovered, is that theory is usually not supported over the immediate short-term, or seconds, not to mention the consideration of structural breaks and the entertaining probability of finding spurious correlations between the Dow Jones Index gaining a few points and Kim Kardashian taking a selfie.
Facebook, on the other hand, and as its CEO has thoroughly described in the congressional hearings, seeks to develop artificial intelligence to identify breaches and inconsistent data on its platform at the highest speed possible. The basis for that determination is the sheer impossible amount of human input required to screen millions, if not billions, of entries within the highest precision in order to not dissatisfy or scare off the “real” users. Does Facebook generate any revenues by implementing such technologies? The simple answer is “no”, aside of their desire to avoid another reputational shakeup which would potentially result in loss of revenue.
This pursuit by Facebook is not very dissimilar to what financial institutions face. Aside of the various regulatory expectations that hit the daily headlines on Bloomberg such as the “Volcker-Rule”, “Dodd-Frank” and “MiFiD” etc, financial institutions are required to implement an ever-more increasing amount of regulatory controls and reporting systems, which unlike Facebook, do not only carry the risk of reputational damage, but also multi-billion dollar penalties in multiple jurisdictions simultaneously. Going beyond the ethical and professional misconduct of staff, penalties arise from failures to detect the simplest of activities. The added revenue of implementing technologies? Keeping the banking license.
The European Anti-Money Laundering directives, which have subsequently been adopted into law or regulatory guidance in the majority of countries have introduced significant additional administrative requirements for financial institutions to combat financial crime and terrorism financing. The essential process in question is known as the “Know Your Client” due diligence exercise, which ultimately aims to force financing institutions and relationship managers to have confidence about the client ownership structure and what particular activities they are involved in, particularly ensuring adherence to sanctions and embargos. Whilst it may be correct to assume that coverage managers “know the client”, the increased pressure to generate revenues, has adversely impacted this. Parallel compliance functions require colleagues to verify the “suitability and appropriateness” of a given product to a client, primarily in the areas of global markets or structured finance. Again, one would assume that there is an implicit understanding, but the financial crises of the 2000s has given all parties a wake-up call.
Many jurisdictions known for tax efficiency are also popular amongst high net-worth individuals or corporations for obscuring the ownership, either as a legitimate protective or tax-saving measure, but at times also to hide the identity of corrupt counterparties, not excluding the so-called “PEPs” or politically exposed persons. The recent “Panama Papers” leak was a perfect example of the lack of understanding amongst banks, who would have rejected establishing a relationship if they had known about the reputational and regulatory consequences, but also the intent of the counterparts.
Establishing a documented “Know Your Client” basis is continued on a risk-adjusted regular cycle, requiring updates upon receipt of new information or just keeping files up-to-date. The most difficult component is for banks to filter through the billions of daily transactions that it carries out, thereby attempting to reject warning indicators with the highest possible degree of confidence. Does this sound familiar? Disqualifying potential financial crime or illegal activity with absolute confidence, or reporting suspect activity whenever they arise is crucial as a single error has dire consequences, leading to penalties or even the revocation of licenses. Filtering according to key words is in itself a very inefficient methodology, as the recent “Russian Laundromat” case may have identified. The mere disguise of a transaction with its included SWIFT parameters needs an identification in regard to where it came from and where it goes to, and whether the transaction fits the “client profile”. I believe this is where the unusual but yet very compatible relationship between economics and artificial intelligence begins.
Economists, with their generalist understanding of market agents and an assumed literacy in statistical techniques, provide the initial steps for any system to recognize statistically significant patterns (see i) perception and ii) recognition). The process, also known as transaction monitoring, is usually enhanced by dynamic rules that adjust based on historical observations. Do historical patterns hold in the future? Not necessarily, but the reactive to proactive transition is where analytics and judgement become important. As models develop and sufficient data is being stored, sophisticated systems are trained to improve themselves to translate and decide, under the assumption they are designed adequately. Basic systems, in comparison, would tend to forward analog flags to the respective officers at the bank. Under consideration of cost pressures and in an avoidance of human errors, our beloved Cobb-Douglas function would dictate that once labor is limited, one must adjust the other parameters.
This is an incredible opportunity that is driving many banks to explore artificial intelligence for entirely new reasons. I never expected to land within the Compliance department of a bank, but alas, I am glad I did! As that is the case, I hereby thank Kim Kardashian for not having taken too many selfies in 2008.
Views expressed in this article reflect the personal view of the author about the subject matter and do not necessarily represent the views of Deutsche Bank AG.
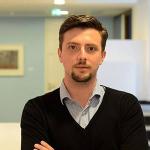
About Firas-Nadim Habach, CFA
Firas-Nadim Habach is currently Country Head of Compliance and AFC Testing in Switzerland at Deutsche Bank and a Doctoral Candidate the Edinburgh Business School
Commenting on his experience at Warwick Economics, Firas stated "It was a challenging course that truly set the stage for life, giving me the optimal understanding and stimulus to engage with and in a globalised, interconnected world"
Are you based in Austria or Switzerland and want to get in touch with the Alumni Community? Contact Firas-Nadim!